Measuring deep learning in educational research
Written by Dr Ian Jones. Ian is a Reader in Mathematics Assessment at the Mathematics Education Centre, Loughborough University. Please see here for more information about Ian and his work.
Quantitative research studies are increasingly valued by researchers, policymakers and teachers, but the findings are only as good as the measures of learning used. It is straightforward to measure some types of learning such as recalling facts and applying algorithms, but we are typically interested in deeper learning, such as understanding of concepts or applying knowledge to novel problems. Unfortunately, deep learning is harder to define and harder to measure, and this inhibits both the quantity and quality of educational research.
To address this problem, researchers at Loughborough investigated efficient methods for producing high-quality measures of deep learning. To do this we adapted and applied measures based on comparative judgement methods. The measures we produced are quite distinct to the traditional tests and scoring rubrics that dominate quantitative studies in educational research. Subject experts are presented with two pieces of student work and asked, simply, which student has demonstrated the deeper learning based on the evidence presented. Many such pairwise decisions from a group of subject experts are collected and then sent to an algorithm to produce a score for each piece of work. The algorithm, based on the Bradley-Terry model, is like a more sophisticated version of calculating points from match results in football. Our comparative judgement-based methods have been shown to be efficient, reliable and valid across a range of target domains and learning contexts.
An example of using comparative judgement to measure deep learning was provided by research led by Dr Ian Jones at Loughborough University. We ran an intervention study in which older primary students were introduced to simple algebra using one of two software packages: Grid Algebraor MiGen. Following the intervention, the main measure was based on an open-ended mathematics prompt as follows.
Explain how letters are used in algebra to someone who has never seen them before. You can use examples and writing to help you give the best explanation that you can.
Students had 10 minutes to complete their answer on a single page. A group of subject experts then made pairwise judgements of students’ responses to the mathematics prompt, and from these decisions we generated a score for each participant. The results showed that students in the Grid Algebra intervention outperformed those in the MiGen intervention.
An example comparison
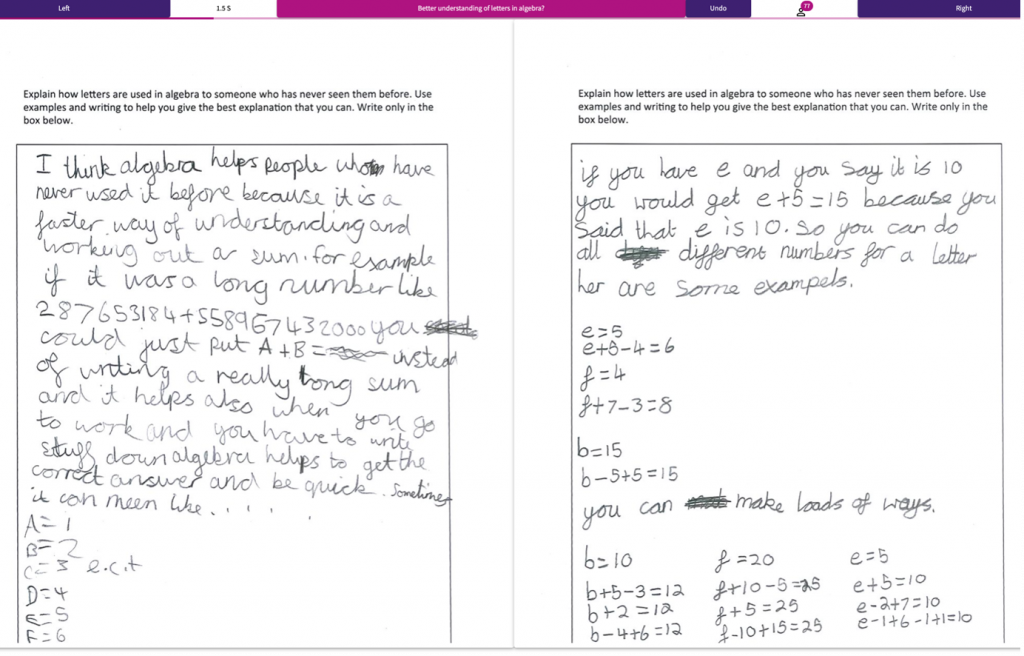
To validate our results, we also administered a standard algebra test that we adapted from the literature. The standard test purports to measure understanding of algebra concepts and so provided a yardstick for our novel comparative judgement-based method. When we conducted the analysis again, but this time using scores from the standard test, we replicated the results produced using scores from the open-ended mathematics prompt. Importantly, the design and implementation of the comparative judgement-based method was far more efficient than the design and implementation of the standard test. Moreover, our approach is flexible and can be readily applied to any target concept without the time and expense required to develop and validate a traditional measure. Therefore, we concluded that comparative judgement-based methods have the potential to improve the quantity and the quality of quantitative educational research studies.
Researchers interested in using comparative judgement methods can do so using the freely available comparative judgement engine at www.nomoremarking.com. We have recently developed a how-to guide for researchers interested in comparative judgement which is available here tinyurl.com/NMM4researchers. You are also welcome to get in touch with Ian at I.Jones@lboro.ac.ukfor further advice and assistance.
Centre for Mathematical Cognition
We write mostly about mathematics education, numerical cognition and general academic life. Our centre’s research is wide-ranging, so there is something for everyone: teachers, researchers and general interest. This blog is managed by Dr Bethany Woollacott, a research associate at the CMC, who edits and typesets all posts. Please email b.woollacott@lboro.ac.uk if you have any feedback or if you would like information about being a guest contributor. We hope you enjoy our blog!